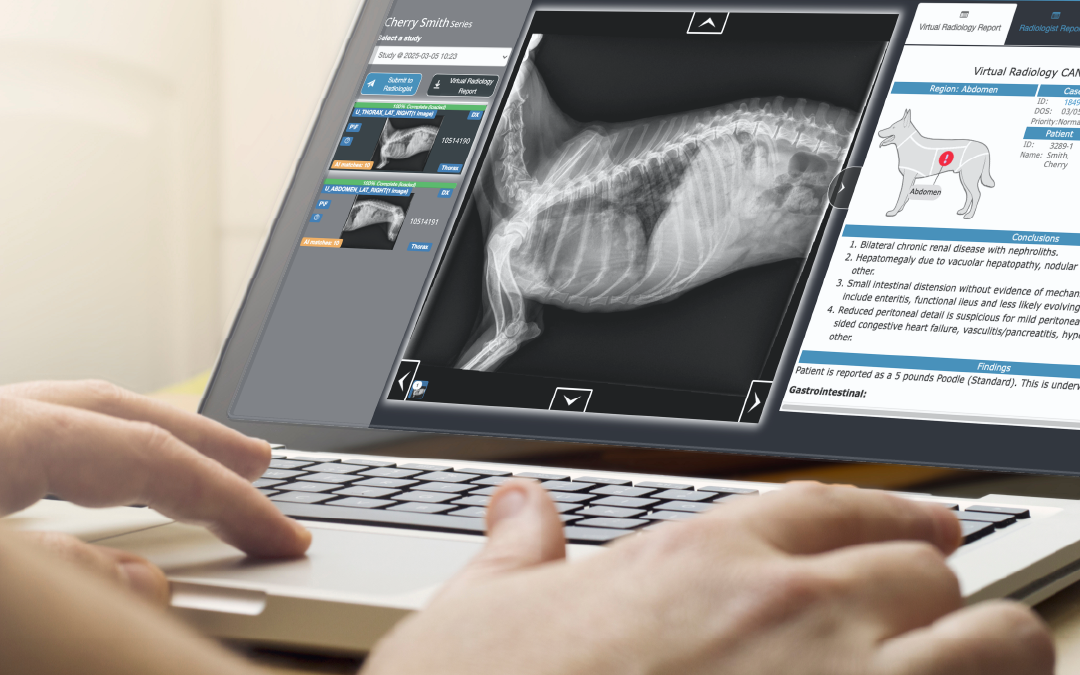
10 Best AI Veterinary Tools (March 2025)
We’re honored to be featured alongside other leading technologies in the veterinary AI space. At Vetology, our goal is to support veterinary patient outcomes.
We’re honored to be featured alongside other leading technologies in the veterinary AI space. At Vetology, our goal is to support veterinary patient outcomes.
In the evolving field of for veterinary imaging, AI classifiers can support an efficient clinical workflow. By understanding how classifiers work, their strengths, and their limitations, veterinarians can make informed decisions about how to incorporate AI into their practice.
The integration of AI technology in our veterinary hospital has transformed the way we approach diagnostics and treatment.
New and improved canine abdominal classifiers expand the range of conditions Vetology’s AI report can screen for.
Vetology’s AI tools for canine and feline X-rays enhance diagnostic accuracy and efficiency, adhering to US FDA Good Machine Learning Practices for reliable results.
Vetology introduces AI-powered feline abdominal radiograph analysis, streamlining diagnostics for veterinary teams.