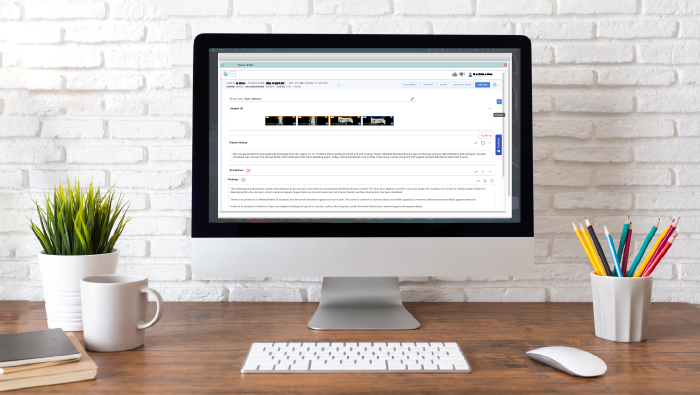
STUDY ABSTRACT – Veterinary Teleradiology Reporting Times
Veterinary Teleradiology Reporting Times: A Retrospective Analysis and Future Directions.
STUDY AUTHORS
Seth Wallack, Andrew Fox, Eric Goldman, Aziz Beguliev, Sommer Aweidah
STUDY PRESENTATION
This study abstract was presented as a poster at the Symposium on Artificial Intelligence in Veterinary Medicine
(SAVY 2.0) in Ithaca, NY | May 16-18, 2025
Background
In human radiology, several metrics are utilized to assess report usefulness, including diagnostic accuracy and Average Time Reporting (ATR). Studies from 2013 and 2017 found reporting times averaged:
- 98 seconds per thorax image,
- 111 seconds per abdomen image
- or ATR of 85 seconds per report, respectively. 1,2,3,4
A study for veterinary ATR for radiographs without and with AI assistance could not be found.5,6
Objectives
- First objective is to baseline ATR for single region veterinary radiograph reporting WITHOUT AI assistance.
- Second objective is to baseline and compare ATR for single region veterinary radiograph reporting WITH AI assistance.
Methods
Case Time Period: January 2023 and April 2025 (28 months).
Case Types: Single region reports were grouped into thorax vs. abdomen and further divided into non-STAT vs. STAT. No distinction was made between typing vs. dictating reports or between canines and felines.
Radiologists: Two US board-certified veterinary radiologists (Drs. Seth Wallack, DACVR and Andrew Fox, DACVR) with between 10-and 20-years experience.
Exclusion Criteria: For the reporting with and without AI assistance, exclusion criteria was cases with ATRs <20 seconds or > 20 minutes.
Platform: Both Radiologists used the patented Vetology® reporting platform featuring personalized generative AI conclusions and recommendations derived from the radiologists’ findings.
AI Generation Time: Separately, average AI generation time was calculated for thoracic and abdominal conclusions and recommendations. Two different findings examples (~200 words and ~300 words) were tested for both thoracic and abdominal AI generation to assess the impact of the findings’ word count on generation time. ATR includes all aspects of report creation from claiming to finalizing each single region case.
Results
WITHOUT AI:
-
- 1696 total cases.
- 1367 used for ATR.
- 329 cases excluded (19%) for >20 minutes.
WITH AI Assistance:
-
- 2489 cases.
- 2280 used for ATR.
- 209 cases excluded (8%) for > 20 minutes.
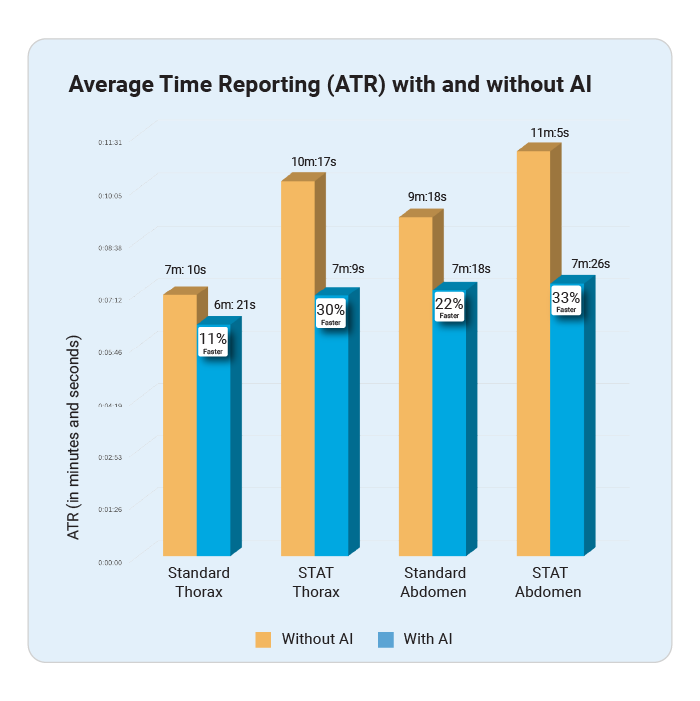
Veterinary Radiologist Average Time Reporting
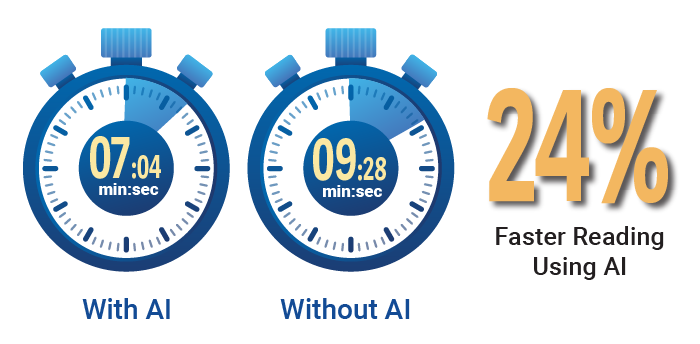
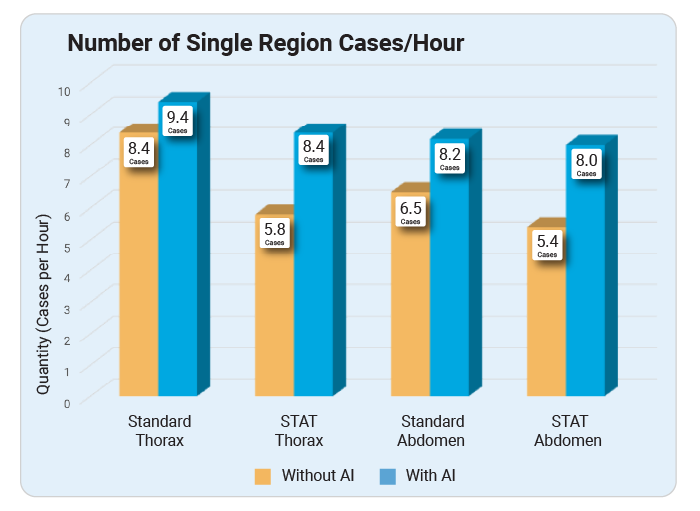
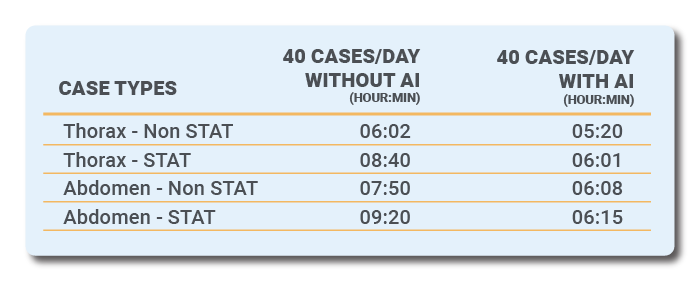
Comparison of Time to Generate Conclusions + Recommendations Using Personalized AI Model
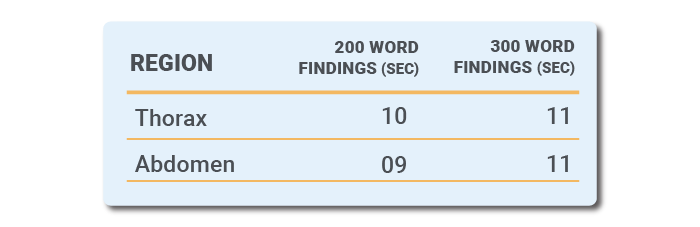
Conclusions
These results reflect a specific veterinary teleradiology company’s baseline ATR with and without AI prelim reporting, recorded at the given points in time. The performance of the platform itself will continue to improve as AI evolves and processing speeds accelerate.
Using the approach described above, this study provides an initial benchmark for veterinary radiology reporting times and suggests that hybrid AI tools may help improve efficiency.
This is a specific veterinary teleradiology company’s baseline ATR with and without AI prelim reporting. This baseline ATR may be comparable to industry-wide ATR for US and European board-certified veterinary radiologists.
This study demonstrates that veterinary radiograph ATR can be reduced by 24% (range 11-33%) using a personalized hybrid generative and visual AI reporting solution. Vetology AI assistance reporting was shown to increase the number of reports per hour from 6.5 to 8.0.
A hybrid model of radiologist-reported findings and Vetology AI-generated, personalized conclusions and recommendations could reduce a 40-case workday by up to 3 hours.
AI-assisted reporting has the potential to reduce radiologist workload fatigue. Generating personalized AI conclusions and recommendations takes seconds.
Funding Disclosures: Vetology Innovations provided funding for this study through salaries.
References
- Cowan IA, MacDonald SL, Floyd RA. Measuring and managing radiologist workload: measuring radiologist reporting times using data from a Radiology Information System. J Med Imaging Radiat Oncol. 2013 Oct;57(5):558-66. doi: 10.1111/1754-9485.12092. Epub 2013 Jul 12. PMID: 24119269.
- Rathnayake S, Nautsch F, Goodman TR, Forman HP, Gunabushanam G. Effect of Radiology Study Flow on Report Turnaround Time. AJR Am J Roentgenol. 2017 Dec;209(6):1308-1311. doi: 10.2214/AJR.17.18282. Epub 2017 Oct 5. PMID: 28981363.
- Boland GW, Halpern EF, Gazelle GS. Radiologist report turnaround time: impact of pay-for-performance measures. AJR Am J Roentgenol. 2010 Sep;195(3):707-11. doi: 10.2214/AJR.09.4164. PMID: 20729450.
- Mityul MI, Gilcrease-Garcia B, Mangano MD, Demertzis JL, Gunn AJ. Radiology Reporting: Current Practices and an Introduction to Patient-Centered Opportunities for Improvement. AJR Am J Roentgenol. 2018 Feb;210(2):376-385. doi: 10.2214/AJR.17.18721. Epub 2017 Nov 15. PMID: 29140114.
- Weissman A, Solano M, Taeymans O, Holmes SP, Jiménez D, Barton B. A SURVEY OF RADIOLOGISTS AND REFERRING VETERINARIANS REGARDING IMAGING REPORTS. Vet Radiol Ultrasound. 2016 Mar-Apr;57(2):124-9. doi: 10.1111/vru.12310. Epub 2015 Dec 17. PMID: 26677167.
- Adams WM. A survey of radiology reporting practices in veterinary teaching hospitals. Vet Radiol Ultrasound. 1998 Sep-Oct;39(5):482-6. doi: 10.1111/j.1740-8261 1998.tb01638.x. PMID: 9771603.