Good Machine Learning Practices (GMLP) Statement
Vetology is committed to advancing veterinary medicine through innovative technologies. Our AI-powered tool for screening canine and feline X-rays exemplifies our dedication to enhancing diagnostic accuracy and efficiency. This statement outlines our adherence to Good Machine Learning Practices (GMLP) across the development, deployment, and maintenance of our AI model.
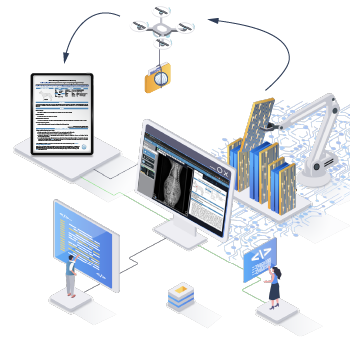
A. Explanation of AI Model Development
Our AI model is developed using a rigorous and systematic process to ensure reliability and clinical relevance.
- Data Collection and Preprocessing: We aggregate a diverse dataset of anonymized canine and feline X-rays from various sources to capture a wide range of anatomical variations and pathological conditions. Data is de-identified in compliance with privacy regulations.
- Annotation and Labeling: Experienced veterinary radiologists annotate the images, providing high-quality labels that serve as ground truth for training. This ensures that the model learns from expert interpretations.
- Model Architecture: We employ state-of-the-art deep learning architectures optimized for image analysis, such as convolutional neural networks (CNNs), to effectively process and interpret radiographic images.
- Training Process: The model is trained using supervised learning techniques with hyperparameter tuning to optimize performance metrics like accuracy, sensitivity, and specificity.
- Bias Mitigation: We actively identify and mitigate potential biases by ensuring balanced representation across species, breeds, ages, and pathological conditions in the training dataset.
B. Explanation of AI Model Pre-release Assurance of Safety and Effectiveness
Before releasing the AI tool, we conduct comprehensive evaluations to ensure it meets safety and efficacy standards.
- Validation Studies: The model undergoes rigorous validation using separate datasets not seen during training to assess its generalizability and robustness.
- Performance Metrics: We evaluate key performance indicators, including accuracy, sensitivity, specificity, and area under the ROC curve (AUC), to quantify the model’s diagnostic capabilities.
- Clinical Testing: Pilot studies are conducted in clinical settings where veterinary professionals use the tool in real-world scenarios to provide feedback on usability and effectiveness.
- Regulatory Compliance: We ensure that our AI tool complies with FDA guidelines for Good Machine Learning Practices.
- Risk Assessment: A thorough risk analysis is performed to identify potential failure modes, and appropriate safeguards are implemented to mitigate any identified risks.
C. Explanation of AI Production Model Deployment and Ongoing Monitoring
Upon deployment, we maintain vigilant oversight of the AI tool’s performance and impact.
- Integration with Existing Systems: The AI tool is seamlessly integrated into veterinary workflows, ensuring minimal disruption and maximal utility.
- User Training and Support: We provide comprehensive training materials and support services to help veterinary professionals effectively utilize the tool.
- Performance Monitoring: Continuous monitoring systems are in place to track the model’s performance in real-time, detecting any deviations from expected outcomes.
- Feedback Mechanisms: We encourage and facilitate user feedback to identify any issues or areas for improvement, fostering a collaborative environment for refinement.
- Data Security: Robust security protocols protect sensitive data during transmission and storage, complying with industry standards for data protection.
D. Explanation of AI Device Ongoing Re-training, Modifications, and Versioning
We recognize the importance of keeping the AI model current with evolving medical knowledge and practices.
- Periodic Re-training: The model is periodically retrained with new data to incorporate the latest findings and address any drift in performance.
- Change Management: Any modifications to the model undergo a structured change management process, including re-validation and documentation.
- Version Control: We maintain strict version control, documenting changes between versions and ensuring traceability of updates.
- Regulatory Updates: Updates to the model are evaluated for regulatory impact, and we ensure continued compliance with applicable regulations.
- Transparency: Users are informed of significant updates or changes to the AI tool, including improvements in performance or functionality.
Conclusion
Vetology is dedicated to providing safe, effective, and reliable AI solutions for veterinary radiology. Through adherence to Good Machine Learning Practices, we strive to enhance diagnostic capabilities while maintaining the highest standards of quality and ethics.